Organizations are investing substantial capital in developing advanced analytics and reporting capabilities that help business users better understand operational challenges and potential risks, ultimately aiming to lower costs and mitigate business risks. However, the continuous development of these capabilities is both time-consuming and costly. As a result, many organizations are intensifying their efforts to leverage emerging technologies, especially Artificial Intelligence (AI), to accelerate progress and stay competitive.
The goal for organizations adopting AI is to enable business users to make natural language queries that deliver accurate, actionable insights in real time. While this may seem like a straightforward task, many organizations continue to rely on the traditional “hamster wheel” approach, where business users must predict their future data needs, triggering cycles of expensive and slow IT development.
The root of the issue lies in the fact that many projects jump into adopting new technologies without first addressing the fundamentals necessary for scalable, long-term enterprise solutions. One of the most critical aspects often overlooked is the need for consistent, high-quality metadata—data that describes other data and provides the necessary context for making sense of it.
Many projects jump into new technologies without addressing fundamentals for long-term solutions.
To address this gap, organizations need to implement a knowledge graph built on a semantic model supported by robust data governance processes. This would establish a unified enterprise metadata layer—a single source of truth and understanding of data across all projects. By contributing to and utilizing this metadata layer, each project can benefit from shared resources, enabling more efficient integration and analytics solutions. This, in turn, reduces the total cost of ownership (TCO) and lays the foundation for impactful AI solutions.
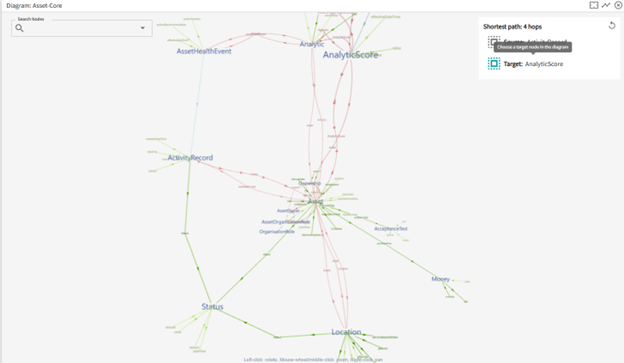
Knowledge graph of metadata
As AI evolves, the integration of knowledge graphs into retrieval and reasoning processes has made metadata management more essential than ever. One such method, Retrieval Augmented Generation (RAG), improves information retrieval by using user inputs to extract relevant data from external or private enterprise data sources. In this case, the enterprise data source is the previously mentioned knowledge graph. The data retrieved from this graph is then combined with the user’s prompt and passed to a large language model (LLM) that adheres to cybersecurity standards.
The integration of knowledge graphs into retrieval and reasoning pipelines has emerged as a critical enabler for context-aware, enterprise-grade AI systems.
This approach empowers the LLM to use both its generalized language capabilities and the organization’s specific data to generate responses that are relevant, accurate, and aligned with the organization’s internal knowledge.
Ultimately, Metadata is the cornerstone of simplifying AI solutions. It provides the context, structure, lineage, and provenance that enables AI systems to understand the relationships between data points, helping avoid the use of outdated, unverified, or incomplete data. Metadata management also streamlines navigation through massive data graphs, reducing redundant computations and ensuring efficiency, accuracy, and scalability. Without proper metadata, AI systems risk becoming confused, biased, or even producing hallucinated results—outputs that are irrelevant or incorrect.
Effective metadata management supports transparency, traceability, and data governance across the AI lifecycle—from data ingestion and feature engineering to model training, deployment, and monitoring.
Robust metadata management enhances data discoverability, mitigates biases, and prevents data drift by ensuring transparency and traceability throughout the AI lifecycle. This enables more accurate, reliable, and effective use of AI, setting the stage for truly transformative, AI-driven business solutions.
SemanticWorx is able to demonstrate how metadata management, semantic modeling, and connected technologies—powered by Affirma—advances AI systems, keeping them context aware, intelligent, compliant, and mostly out of trouble.
Learn more about Affirma and how to navigate change! Speak to a member of the SemanticWorx team.