Utilities manage complex assets across electrical grids and water distribution systems, necessitating accurate tracking and maintenance. However, fragmented data systems, lack of integration, and inconsistent information lead to inefficiencies, increased risks, and diminished performance.
Without a unified data strategy, decision-making becomes difficult, impacting asset management and compromising network reliability. Effectively implementing enterprise information management across their technology ecosystem remains a significant challenge.
Multiple siloed data sources
Inconsistent terminology and data structures across systems and departments create confusion and data silos, impacting stakeholders and operations. These fragmented data sources underscore the need for a unified enterprise data vocabulary and standardized processes.
The presence of siloed data emphasizes the importance of a unified approach.
Without standardization, organizations face miscommunication, inefficiencies, increased errors, and difficulties in sharing and integrating information across the enterprise.
Data quality issues
Incomplete or inaccurate data undermines decision-making, asset maintenance, and network performance. Missing or erroneous data increases operational risks, such as unexpected failures or misinformed forecasts. Addressing data quality is crucial for informed decisions and for enhancing the reliability of operations.
Weak data foundation for analytics
The lack of a unified, well-structured data foundation poses a major challenge, preventing the full utilization of advanced analytics for informed decision-making. Fragmented information hinders effective analysis, predictive modeling, and reporting, limiting the ability to generate actionable insights and optimize operations.
Limited interoperability
In-house applications often operate in silos, lacking interoperability and making data integration and sharing across systems challenging. Manual, application-specific processes—such as spreadsheet manipulation and custom scripts—further complicate workflows, creating a fragile and inefficient system. Over time, these issues become increasingly unmanageable, especially as personnel changes disrupt continuity and exacerbate challenges. A unified data strategy is essential to streamline processes, enhance data control, and ensure long-term sustainability.
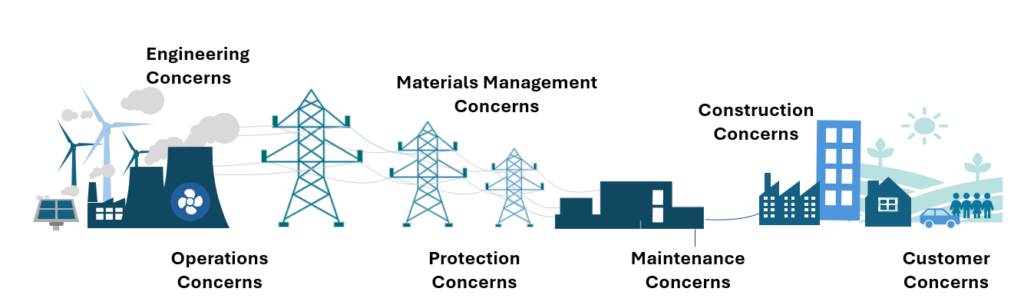
Asset perspectives across the data lifecycle
Unlocking the power of Affirma
Affirma facilitates the curation and management of an Enterprise Semantic Model (ESM), creating a unified vocabulary across the enterprise ecosystem. By standardizing language and data definitions, it enhances consistency and alignment, fostering better communication and seamless integration across the enterprise.
Leveraging industry standard models
A critical first step in managing data across an organization is to create a shared understanding of key concepts and entities. Affirma enables the integration of industry-standard models, such as the CIM (Common Information Model), into its data catalog. By starting with these established models, organizations can leverage widely recognized frameworks that are already accepted within the industry. These models provide a standardized foundation for structuring data, eliminating the need to reinvent the wheel. Aligning with these standards ensures interoperability with other systems, facilitating seamless integration both within the organization and with other industry players.
By grounding the approach in proven models, organizations can align with trusted frameworks embraced across the industry.
The Common Information Model (CIM) is a standardized model used in the utility industry to represent and exchange data related to electrical grids and systems. It is maintained by the CIM User Group (CIM UG) and implemented as various standards by the International Electrotechnical Commission (IEC). These standards include IEC 61970 - Grid, IEC 61968 - System Integration, IEC 61850 - Automation, and IEC 62325 - Markets.
Capitalize on applications as reference models
Each application within an organization typically has its own unique data representations and interfaces. By integrating these into the Affirma data catalog, an organization can align the application-specific vocabulary with the canonical vocabulary in the ESM. This alignment fosters consistent data exchange and integration, ensuring smooth communication across systems.
Curate an Enterprise Semantic Model to represent enterprise vocabulary
Creating an Enterprise Semantic Model (ESM) is crucial for resolving inconsistencies in terminology across the organization. The ESM serves as a centralized, curated representation of the organization’s vocabulary, capturing the meanings and relationships of terms used across departments and systems. To build the ESM, you can leverage existing industry or application models imported into Affirma and create new object definitions. By developing and maintaining the ESM, organizations can eliminate confusion, ensure consistency, and enhance communication across teams. Additionally, the ESM establishes a solid foundation for data governance, ensuring consistent interpretation of data, regardless of the system or user involved.
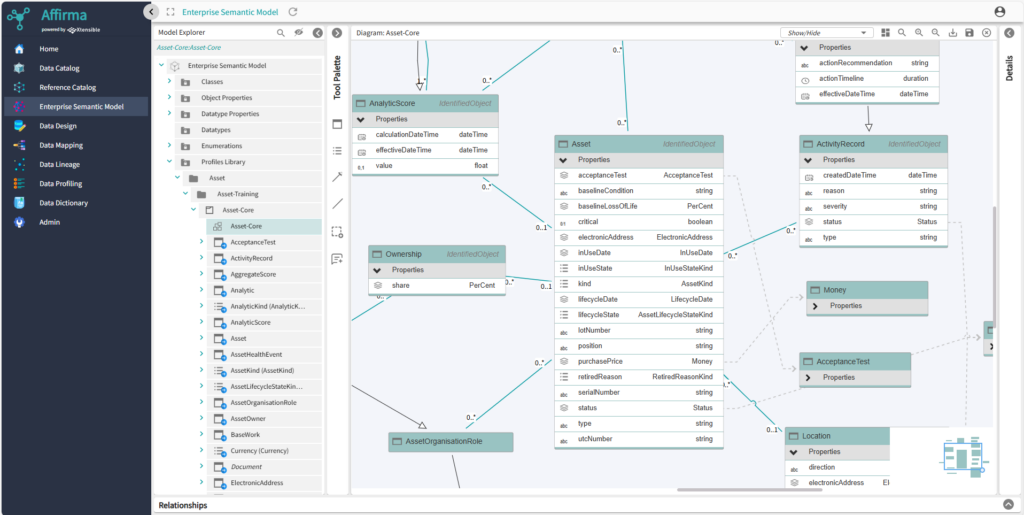
Enterprise Semantic Model
Generate canonical interface specifications based on enterprise vocabulary
With a well-defined Enterprise Semantic Model in place, you can leverage Affirma to create canonical interface specifications—standardized, precise definitions of how data should be represented and exchanged between systems. These specifications are based on the organization's established vocabulary within the ESM, ensuring that every interface across applications communicates using a unified language. This approach simplifies the complexities of previous point-to-point integrations and reduces system brittleness, as new integrations can be built around the common vocabulary rather than relying on application-specific proprietary interfaces.
Track Data Movements using Data lineage
Affirma provides advanced data lineage capabilities that allow organizations to visualize and understand the flow of data, offering a clear view of the provenance of both data and metadata.
Data lineage helps monitor data quality and integrity by tracing issues across the data lifecycle.
This enables more effective monitoring of data quality and integrity, helping to pinpoint issues within the data lifecycle. Additionally, data lineage enhances regulatory compliance, auditing, and troubleshooting by offering detailed documentation of how and where data has evolved over time.
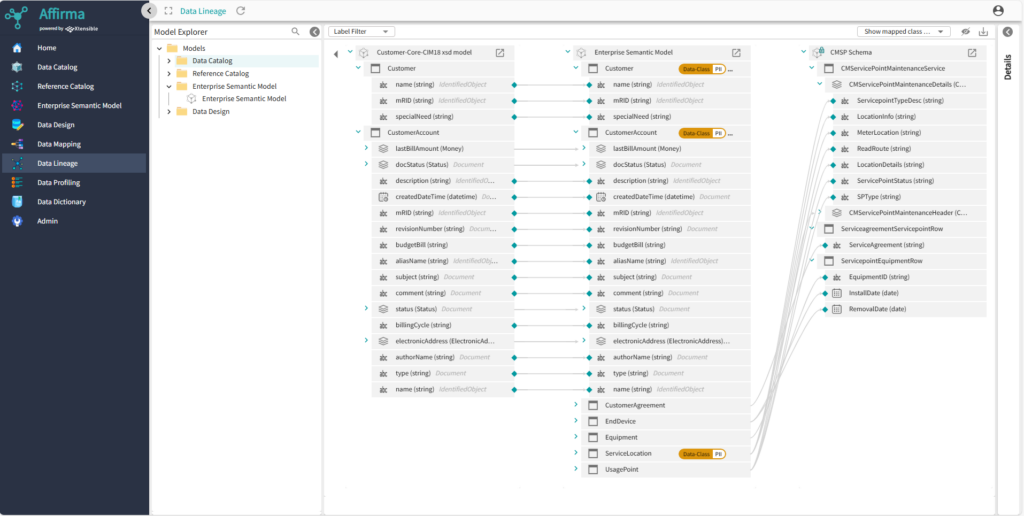
Data Lineage
Improve Data Quality using Data Profiling
Affirma can analyze data stores and generate detailed reports on data anomalies and patterns caused by fragmented data processes and manual data entry errors. These reports identify potential data gaps and inaccuracies, offering insights into the scope and impact of each issue, helping the organization prioritize corrective actions more efficiently.
Maximizing impact with Affirma
By leveraging Affirma, internal data processes and data quality would be greatly improved. A canonical Enterprise Service Model (ESM) would serve as the single source of truth across the organization, streamlining the vocabulary used by both employees and applications. A comprehensive data governance framework would be implemented to manage enterprise data flows, formalizing and standardizing change management processes, resulting in enhanced efficiency and control.
Furthermore, the organization would be empowered to develop an API service platform based on the canonical API definitions generated by Affirma. Since these APIs would be built around the canonical ESM objects, both services and applications would communicate using a unified enterprise vocabulary, boosting consistency and operational efficiency.
Finally, data quality would be improved through Affirma’s data profiling reports, allowing for quick identification and correction of missing or erroneous data.
Envisioning the future
While Affirma has already made significant progress in enhancing the consistency, quality, and governance of enterprise data and processes, our vision for the future promise even greater improvements.
AI-Driven Instance Data Mapping and Inference to ESM
Affirma will utilize the knowledge graph of the metamodel and AI-powered machine learning inference algorithms to automatically map instance data to the Enterprise Semantic Model (ESM). This automation drastically reduces the time spent reconciling different data formats across systems during integration, streamlining validation and testing workflows while enhancing overall efficiency.
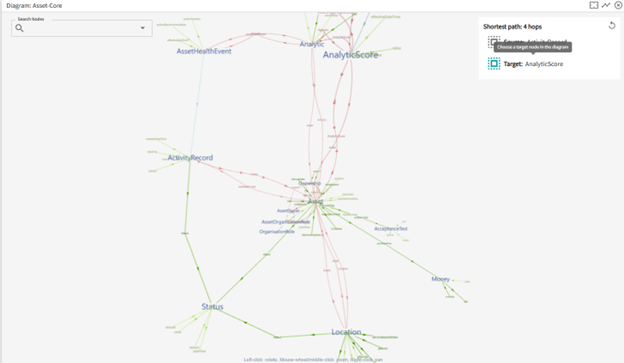
Knowledge graph of metadata
ESM-Based Reasoning for Identifying Issues in the Instance Data
Another advanced feature of Affirma will enhance the instance-to-ESM mapping by leveraging the ESM metamodel to conduct comprehensive data quality checks on instance data. This improved reasoning capability will then automatically detect complex issues, such as asset lifecycle management, predictive maintenance, network troubleshooting, demand forecasting, and energy efficiency analysis, significantly boosting efficiency, reliability, and data quality across the enterprise.
Learn more about Affirma and how to navigate change! Speak to a member of the SemanticWorx team.